New machine-learning approach to discover hidden geothermal signatures
Discovery of hidden geothermal resources is challenging. Carbon Solutions scientists have developed a new machine learning method to meet this challenge. It requires the mining of large datasets with diverse data attributes representing subsurface hydrogeological and geothermal conditions. The research demonstrates an approach based on machine learning to process a geothermal dataset from southwest New Mexico (SWNM). The study region includes low- and medium-temperature hydrothermal systems. Several of these systems are not well characterized because of insufficient existing data and limited past explorative work. This study discovers hidden patterns and relations in the SWNM geothermal dataset to improve our understanding of the regional hydrothermal conditions and energy-production favorability. This understanding is obtained by applying an unsupervised ML algorithm based on non-negative matrix factorization coupled with customized k-means clustering (NMFk). NMFk is a part of the SmartTensors ML framework (https://github.com/SmartTensors). It automatically identifies (1) hidden signatures characterizing analyzed datasets, (2) the optimal number of these signatures, (3) the dominant data attributes associated with each signature, and (4) the spatial distribution of the extracted signatures. The resulting ML model can be applied to predict geothermal conditions and their uncertainties at new SWNM locations based on limited data from unexplored regions.
50-day open-access link to the paper: https://www.sciencedirect.com/science/article/pii/S037565052200222X
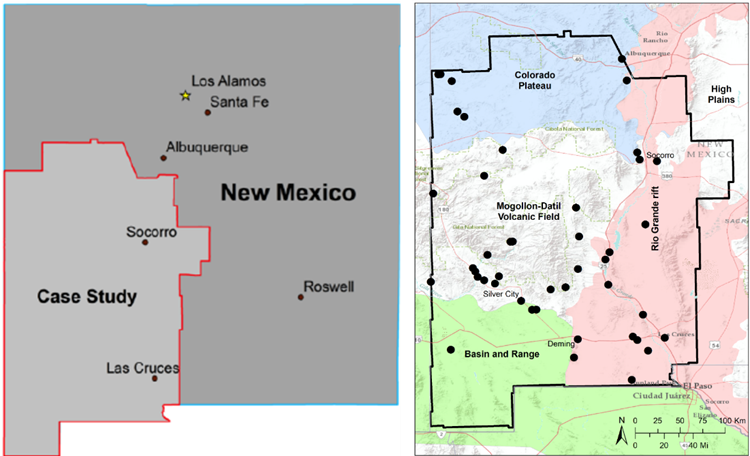